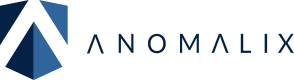
Cyber attacks have become more sophisticated, more common, and less predictable. As businesses expand their online presence, they also increase their vulnerabilities—across networks, devices, and user identities.
Legacy security controls, though still useful, are designed for known threats and static rule sets. That approach creates gaps when attackers exploit unknown vulnerabilities or sidestep pre-defined controls. Detection often comes too late, after the damage has been done.
Machine learning and artificial intelligence are revolutionizing security responses. Such systems analyze huge amounts of information in real time, detect strange patterns, and adapt to new methods without requiring constant manual updates. The result is a more nimble, data-driven approach to cybersecurity—one that aims to detect threats earlier and facilitate faster, better-informed decision-making.
For businesses with ever-growing demands to protect systems, information, and people—and reduce the risk of identity-related breaches—AI is not an option. It's becoming indispensable.
Cybersecurity is no longer about responding to singular incidents—it's about coping with a steady stream of activity across distributed systems, users, and endpoints. The volume of data moving around enterprise networks makes it greatly difficult to monitor manually. Even automated systems with static rules struggle to keep up with the new techniques attackers use.
Artificial intelligence overcomes these challenges by doing what humans and rule-based tools cannot do: analyzing massive, complex datasets at once, and spotting subtle anomalies that may signal an attack. Instead of looking for known signatures, AI models look for behavioral patterns—such as a user logging into unknown systems at strange hours or a device behaving differently than usual.
These models improve over time, learning from false alarms and confirmed threats. The more data the system is trained on, the better it becomes at separating actual issues from noise. This shift in approach is one of the most important ways AI is transforming cybersecurity. It brings speed, scale, and flexibility to threat detection and decision-making processes, enabling teams to prioritize what matters most.
Cyber attackers usually move in patterns that are difficult for traditional systems to detect. An attacker can jump horizontally between networks, gain access to sensitive data gradually, or exploit credentials without triggering any alarms. Machine learning improves cyber threat detection by finding hidden patterns in user behavior, system behavior, or network behavior that would not otherwise be seen.
Instead of relying on pre-programmed rules, AI models are taught to learn from large volumes of data that allow them to recognize patterns of bad behavior even when those patterns are not recognized. This allows security teams to spot suspicious behavior more rapidly, often before an attack can do damage.
One of the strengths of machine learning in cyber threat detection is that it can identify patterns rather than particular threats. Signature-based tools might fail to detect new variants of malware or unknown vulnerabilities, whereas AI systems are more interested in behavior—like strange file transfers, unexpected login points, or strange communication patterns between devices.
By modeling what "normal" appears as across a network, AI can recognize when something goes beyond that, even if the exact tactic or technique applied has never been witnessed before.
Endpoints—laptops, mobile phones, IoT devices, and remote workstations—are now prime targets for attackers. Every device connected to a network is a potential point of entry for a cyber attack. Protecting endpoints effectively involves monitoring a large number of devices, each with its own usage patterns and threats.
AI endpoint protection seeks to detect the earliest signs of compromise. This could be sudden resource spikes, illegal installation of programs, unexpected file modifications, and so on. Once this is discovered, AI can quarantine the affected devices, isolate the malware, and alert security teams without giving the attacker enough time to go further.
With network-level threat detection combined with AI-driven endpoint security, businesses can establish a stronger, more resilient cybersecurity foundation—one that can respond to threats as they occur, not after the fact.
Learn more about aligning AI with your identity strategy.
User identities and access controls have always been a central component of cybersecurity. Yet in today's environments—where remote workers, contractors, vendors, and cloud services are becoming more common—traditional identity and access management (IAM) tools are pushed to their limits. Static access policies and the occasional audit can’t keep up with changing behavior and threats.
AI for identity and access management introduces a dynamic element to security. Rather than relying on role-based permissions or periodic audits, AI monitors user activity continuously and flags deviations from normal activity patterns.
AI-based IAM solutions track login trends, access timings, device activity, and activity patterns to develop a baseline for each user. When a user behaves out of the ordinary—such as logging into sensitive systems outside work timings or downloading abnormal amounts of data—the system is able to require additional authentication protocols or restrict access automatically.
Continuous authentication using behavioral factors reduces reliance on static passwords or periodic verification checks. Instead, users are authenticated passively and based on how they interact with systems, making it harder for attackers to abuse credentials even when they are breached.
Third-party users, contractors, and suppliers usually need access to internal systems but this brings added risk. AI in IAM helps to monitor external accounts and privileged users in real-time, enforcing stricter controls where necessary. Proactive monitoring facilitates Zero Trust initiatives by never trusting and always verifying.
By integrating AI into identity management processes, organizations approach a pattern in which access is constantly reassessed and remastered in line with real-world actions rather than static presumptions.
Most cybersecurity defenses are reactive—they find threats only after they've already infiltrated a network. AI-powered cybersecurity defense systems aim to push that timeline ahead. Instead of simply responding to attacks, machine learning models make it possible to anticipate where vulnerabilities are likely to be exploited and allow organizations to act before breaches occur.
Predictive models consume historical information, real-time network traffic, user behavior, and threat intelligence feeds to forecast probable risks. By identifying patterns that typically precede security incidents, AI can recommend actions like patching specific vulnerabilities, restricting high-risk access, or enhancing monitoring in specific zones.
Not all threats are equal. Certain vulnerabilities are more risky than others based on how simple they may be to exploit or how badly they may damage. Machine learning helps security teams prioritize by applying risk scores to users, systems, and potential incidents. Instead of overwhelming analysts with an endless stream of alerts, AI determines where attention is most urgently needed.
This risk-based approach allows organizations to more efficiently manage resources, focusing on the most significant issues rather than reacting to every minor anomaly.
AI does not replace human judgment but offers security teams earlier warning, better context, and faster analysis. By integrating predictive models into cybersecurity routines, organizations can transition from a defensive to a proactive position—anticipating problems and hardening defenses before attackers even have a chance to act.
AI-powered systems can generate a large volume of false alarms, especially if models are not accurately tuned. False positives—legitimate activity incorrectly flagged as suspicious—can overwhelm security teams and destroy trust in automated tools. In some cases, this leads to threat gaps when real issues are lost in noise.
AI models are only as good as the data they are trained on. If the training data is bad, outdated, or biased, the system will not be able to detect threats properly. In cybersecurity, attack mechanisms continue to evolve, and behavior patterns vary by industry and organization.
Most systems for AI are not extremely transparent. The security team will receive a risk score or alert but will not necessarily know precisely how the system came to its conclusion. This unexplainability can lead to challenges in decision-making and compliance, particularly within regulated enterprises.
Attackers are learning to exploit AI models themselves. Adversarial attacks, for instance, whereby attackers feed fake information into training data or manipulate inputs, can deceive AI systems or hide malicious activity. Such threats highlight the need for continuous testing and monitoring of AI-powered tools.
More industries are creating industry-specific models as AI usage continues to grow. A bank can train systems to watch for aberrant transaction activity, while a medical firm will focus on safeguarding patient data. The additional accuracy is derived by using the specific behavior and threat profiles of each environment.
Artificial intelligence is being increasingly paired with automation tools within Security Orchestration, Automation, and Response (SOAR) systems. These technologies can provide faster, more uniform reactions to repeated threats—such as isolating infected systems or resetting passwords—without needing to wait for human intervention.
As more privacy issues arise, federated learning is gaining momentum. The approach allows AI models to be trained somewhere else or on another device without exposing sensitive information to a central server. It provides better protection of privacy without allowing collective threat intelligence.
Artificial intelligence and machine learning are revolutionizing how organizations approach cybersecurity. Through enabling faster identification of threats, bolstering endpoint security, strengthening identity management, and supporting proactive defense strategies, AI-based solutions offer a smarter, more responsive style of risk management.
On the other side, corporations should also become aware of the limitations and challenges implied by such technologies. Suitable introduction, regular oversight, and manual interference are just as important as the technologies themselves.
As threats become more sophisticated, the role of AI in cybersecurity will only grow—allowing businesses to remain one step ahead, respond more rapidly, and create more robust digital defenses.
Machine learning and AI are useful tools, but their usefulness is only as good as their integration with your existing security infrastructure. To leverage identity and access management, endpoint security, and predictive defense, success depends on the right mix of technology, expertise, and ongoing monitoring.
Want to know how AI can be a component of your cybersecurity? Reach out to us at info@anomalix.com to find out how Anomalix can help you use scalable, practical AI-based solutions for your enterprise.